Evaluating Accession Decisions in Customs Unions: A Dynamic Machine Learning Approach
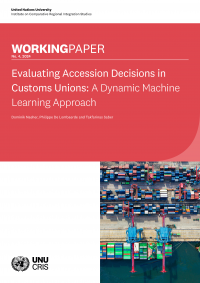
Previous work in the literature on regional economic integration has proposed the use of machine learning algorithms to evaluate the composition of customs unions; specifically, to estimate the degree to which customs unions match ‘natural markets’ arising from trade flow data or appear to be driven by other factors such as political considerations. This paper expands upon the static approaches used in previous studies to develop a dynamic framework that allows to evaluate not only the composition of customs unions at a given point in time, but also changes in the composition over time resulting from accessions of new member states. We then apply the dynamic algorithm to evaluate the evolution of the global landscape of customs unions using data on bilateral trade flows of 200 countries from 1958 to 2018. The analysis generates a rich set of insights on the forces underlying each accession round of the European Union and those of other customs unions in the world, complementing the insights obtained from static analyses.