Unlocking Solutions: AI For Disaster Management and Psychosocial Well-being
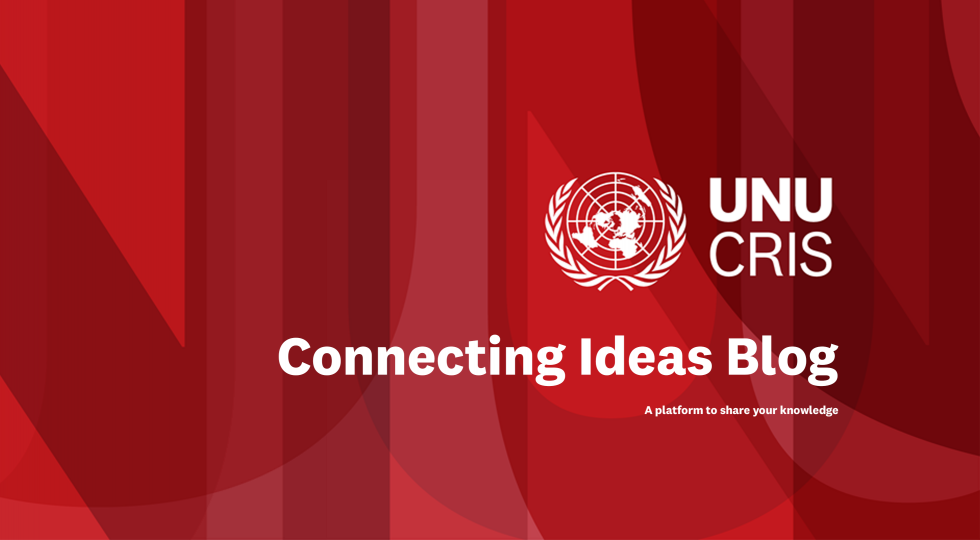
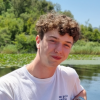
Research Intern, UNU-CRIS
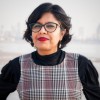
Senior Research Fellow
18 September 2023 | #23.07 | The views expressed in this post are those of the author(s) and may not reflect those of UNU-CRIS.
Artificial Intelligence (AI) has emerged as a powerful tool in various fields over the last few years. Thanks to improvements in algorithms, a rise in computational power, and the accessibility to an avalanche of data, machine learning techniques have become commonplace in industries like finance or medicine. However, recently interest has also grown in leveraging AI techniques in mitigating the devastating impact of natural calamities. With its potential to enhance prediction, response, and recovery efforts, AI offers not only a way to reinforce natural disaster management during the ongoing event, but also to alleviate some of the mental health conditions and support the psychosocial well-being of affected citizens, survivors, and exposed responders. The latter is especially interesting in light of the ever-increasing global prevalence of mental health conditions, principally in burgeoning climate crises where the need for accessible and effective interventions remains pertinent.
In this blog, we provide vital insights into how AI can offer new avenues for gaining critical insights into mapping and addressing disaster impact, enabling the effective deployment of early warning systems to alert vulnerable communities, and understanding, diagnosing, and supporting Mental Health and Psychosocial well-being needs, mainly in climate crisis settings and disaster events.
AI and Disaster risk assessment and management
As disruptive technologies such as artificial intelligence emerge, new potential for enhancing disaster resilience and risk reduction are being addressed. AI-driven models are increasingly proving successful for earthquake and flood monitoring. One example is the M-LARGE project, where scientists combined satellite images with deep learning models in order to provide an early warning signal before strong shaking and tsunami onsets. This innovation consistently outperformed conventional methods, improving predictions in previously affected areas. Another example is the use of neural networks in urban flood nowcasting, referring to the near-future prediction of flood status in the wake of extreme weather unfolding. Here, researchers used community features, such as tweets, 311 calls etc. to model /predict the unfolding of a flooding event. This helps the community to anticipate risks and impacts and can create situational awareness.
Along with the set of merits AI is offering, Kuglitsch et al., address the limitations of this new technology, stating that it remains crucial in realising its benefits.
Like any tool, the effectiveness of an AI model depends on the information it's built upon. Therefore, data quantity is the first challenge. In disaster situations, the focus often leans towards the 'response and recovery' phase. However, a crucial challenge lies in gathering sufficient enough data on different aspects. Even though the frequency of disasters has increased over the last few years, their occurrence is still relatively rare. This limits the amount of data available for training AI-based models, thus restricting their development and generalizability. Second, there is the problem of data quality. Here, technical factors like calibration, spatiotemporal resolution, and independency come into play. A third problem has little to do with the actual models but their implementation. When it comes to using AI to tackle disasters, an important piece of the puzzle needs attention. You see, AI algorithms are usually crafted by experts in universities or labs. They create these tools that can make a real difference. But here's the catch – once they're done, the results often end up in conferences, research papers, or committee meetings. They might not reach the people who can actually put them to use in the real world – like governments and decision-makers. That's a challenge we need to overcome.
AI for Mental healthcare and personalised support
In the first section, we reflect on AI's potential to prevent casualties during disasters through better prediction and management. Despite that, events like floods, hurricanes or earthquakes not only affect the community on a physical level; Mental health problems like depressive disorders, anxiety and related disorders have become increasingly common in our society and are affecting the population’s health in multiple ways. While the impact of AI on handling psychosocial problems in a climate crisis setting remains relatively unexplored, its potential to enhance psychological well-being in general has caught the attention of media, scientists, and more. Researchers like Shatte et al. (2019) have delved into this realm, examining over 300 papers. Their findings shed light on how Machine Learning can be used in mental health, highlighting four key areas of application shown in Figure 1.
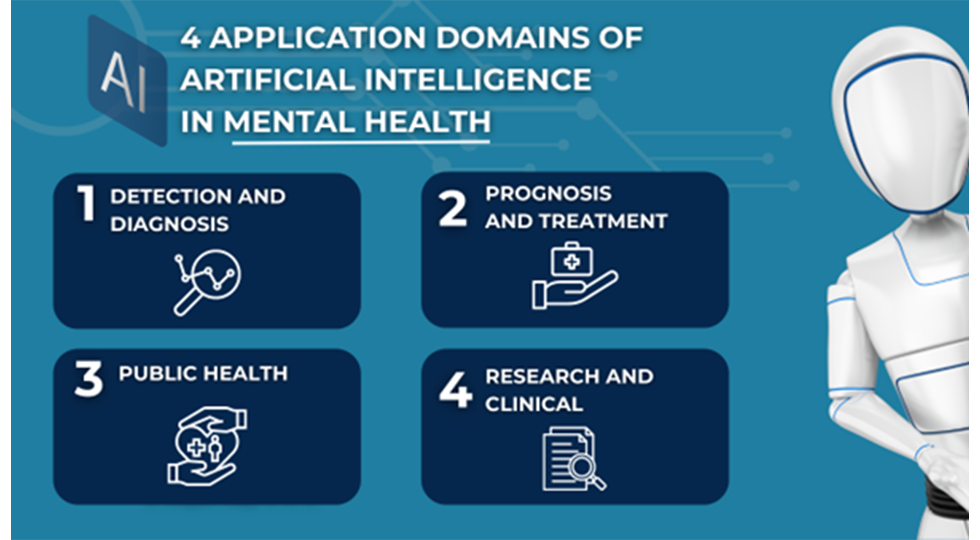
Fig. 1 The four application domains of artificial intelligence in mental healthcare adapted from Shatte et al., (2019)
Within the realm of disaster management, the aspect of public health holds a key yet underexplored domain. By observing the mental well-being of a community in the aftermath of a disaster event, we can gain valuable insights into the multifaceted human impacts of extreme climate settings. And here's where AI steps in, paving the way for smarter health system responses. Take a look at this example: scientists use AI to analyse electronic health records. By doing so, they're predicting suicide risks just as accurately as human experts. That's some promising potential! But there's more: Let’s think about the power of social media combined with AI. Researchers Saha Choudhury actually figured out how to gauge stress levels in college students after they experienced gun violence. This technique could be the key to understanding the mental toll of climate crises and disasters in the short and long run.
And here's another critical point: AI might be able to support mental health diagnoses by analysing speech patterns, facial expressions, and behaviours to spot mental health conditions. This could cut down on errors in diagnosis, boost treatment results, and eventually aid in better resource allocation. So, when disaster or climate crisis strikes, AI could be our tool in shaping resilient societies with better mental healthcare options, amplified climate services, and inclusive disaster risk response delivery systems.
Way forward
We reiterate that the critical strength of AI lies in its ability to analyse vast amounts of data quickly and identify patterns from large data sets and settings. In mental health, AI can analyse data from multiple sources, such as electronic health records, social media platforms, and wearable devices, to detect trends, patterns, and correlations and help identify at-risk individuals. We summarise the five key points (Figure 2) and insights that hold the potential to provide actors, agencies and institutions with the appropriate knowledge to better assist vulnerable individuals after a traumatic event and to make optimal use of scarce resources.
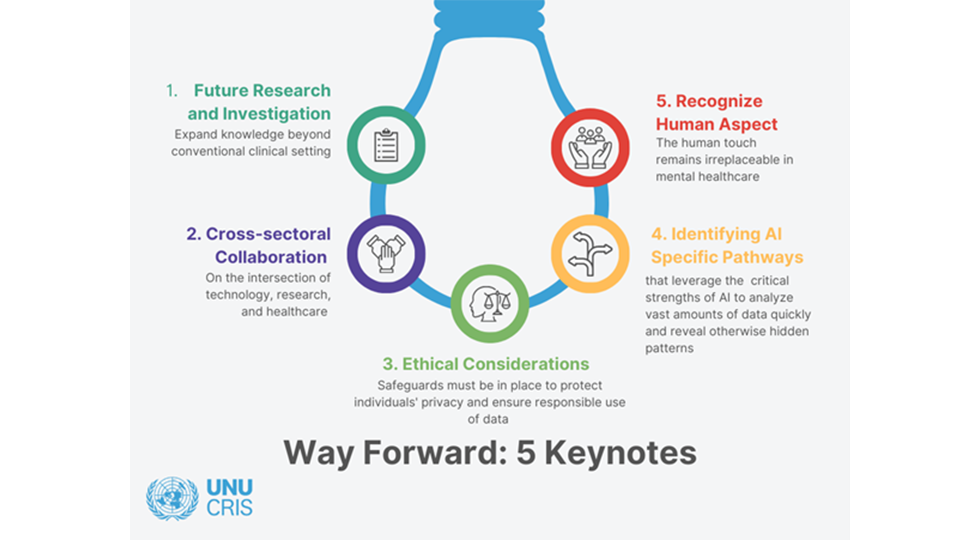
Fig 2. Ways forward: five keynotes for the future of AI
First, despite the current limitations due to the scarcity of research in this area, AI’s (machine learning methods) potential for future investigations to capitalise on advancements and leverage indicators derived from social media and electronic health records remains promising. Current AI applications in mental health mainly focus on mental disorders like schizophrenia and other psychotic disorders. This indicates a significant gap in our understanding of how they can be used to study other mental health conditions.
Second, in the context of mental health, enhanced cross-sectoral collaboration is vital to ensure the successful integration of new technologies for the benefit of public health programs and policies. The intersection holds significant promise for advancing mental health diagnosis, treatment, and support.
Third, addressing challenges like ethical considerations and data privacy should also be considered when implementing AI in mental health scenarios. Safeguards must be in place to protect individuals' privacy and ensure responsible use of data. Establishing transparent and accountable practices for data collection, storage, and sharing is crucial to building trust and maintaining the confidentiality and security of sensitive information.
Fourth, identifying pathways to leverage the critical strengths of AI that lie in its ability to analyse vast amounts of data quickly and identify patterns that might be challenging (or time-consuming) for humans to discern. In mental health, AI can analyse data from multiple sources, such as electronic health records, social media platforms, and wearable devices, to detect trends, patterns, and correlations and help identify at-risk individuals. This enables timely interventions and personalised support services, alleviating the burden on healthcare professionals.
Fifth, while AI offers exciting possibilities in supporting mental health and psychosocial well-being, we must remember that it should complement, not replace, human care. The human touch, empathy, and therapeutic alliance remain irreplaceable in mental healthcare. AI should be seen as a tool to augment and enhance mental health services, empowering clinicians and individuals with valuable insights and resources.
It is fair to say that AI holds the potential to support disaster risk reduction and related mental healthcare. By leveraging its unique characteristics, we envision a future where climate services are better integrated, more effective, and tailored to individual or community needs and sustainability goals (see Annexure 1). Yet, as we venture into this crossroads of AI and mental health, let's tread carefully. As AI innovations transform into tangible solutions, the vision should strike a balance between technological advancements and human connections, ensuring that the well-being and dignity of individuals and communities remain at the core of understanding, designing, and applying solutions.
UN Sustainable Development Goals AI is enabling in the context of natural disasters:
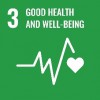
Target 3: Ensure healthy lives and promote well-being for all at all ages.
- In mental healthcare, AI can analyse data from multiple sources, such as electronic health records, social media platforms, and wearable devices, to detect trends, patterns, and correlations. This way, AI algorithms could help identify at-risk individuals, and timely interventions and support services can be provided.
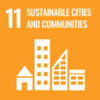
Target 11.5: By 2030, significantly reduce the number of deaths and the number of people affected and substantially decrease the direct economic losses relative to global gross domestic product caused by disasters, including water-related disasters, with a focus on protecting the poor and people in vulnerable situations
- By incorporating AI models in natural disaster management, extreme weather events can be better predicted as they unfold, thereby creating more situational awareness, which could decrease casualties and economic losses.
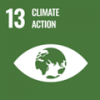
Target 13.1: Strengthen resilience and adaptive capacity to climate-related hazards and natural disasters in all countries
- The improved prediction of climate-related hazards improves resilience and preparedness in disaster management.